Assessing Verbal Eyewitness Confidence Statements Using Natural Language Processing
Document Type
Article
Publication Date
3-2024
Abstract
After an eyewitness completes a lineup, officers are advised to ask witnesses how confident they are in their identification. While researchers in the lab typically study eyewitness confidence numerically, confidence in the field is primarily gathered verbally. In the current study, we used a natural language processing approach to develop an automated model to classify verbal eyewitness confidence statements. Across a variety of stimulus materials and witnessing conditions, our model correctly classified adult witnesses’ (N = 4,541) level of confidence (i.e., high, medium, or low) 71% of the time. Confidence-accuracy calibration curves demonstrate that the model’s confidence classification performs similarly in predicting eyewitness accuracy compared to witnesses’ self-reported numeric confidence. Our model also furnishes a new metric, confidence entropy, that measures the vagueness of witnesses’ confidence statements and provides independent information about eyewitness accuracy. These results have implications for how empirical scientists collect confidence data and how police interpret eyewitness confidence statements.
Keywords
eyewitness confidence, verbal confidence, natural language processing, eyewitness accuracy, eyewitness misidentifications, police officers, machine learning approaches, Transformer-based Large Language Mode
Publication Title
Psychological Science
Repository Citation
Greenspan, Rachel Leigh; Heaton, Paul; and Lyman, Alex, "Assessing Verbal Eyewitness Confidence Statements Using Natural Language Processing" (2024). Articles. 410.
https://scholarship.law.upenn.edu/faculty_articles/410
https://doi.org/10.1177/09567976241229028
DOI
https://doi.org/10.1177/09567976241229028
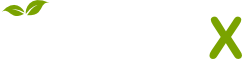
- Citations
- Citation Indexes: 5
- Usage
- Abstract Views: 39
- Captures
- Readers: 7
- Mentions
- News Mentions: 2